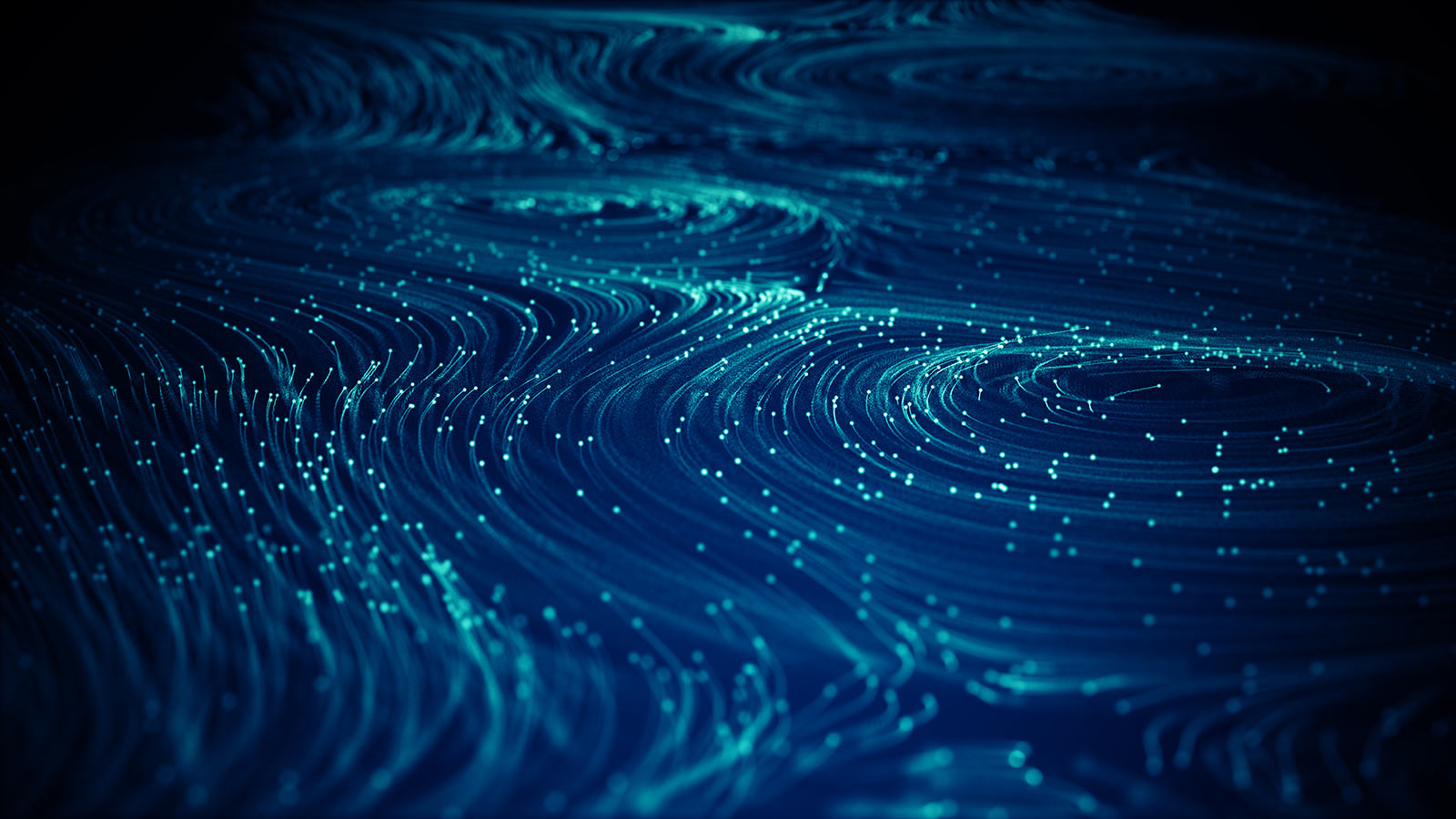
What's inside?
Businesses reliant on the protection of proprietary and sensitive information are at risk from AI’s indiscriminate ingestion of data. Data has become a raw material that feeds into GenAI foundation model training, and its quality and quantity determine the utility of an AI. The true value of GenAI is in its ability to provide high-quality outputs that are based on a holistic view of data and information it has ingested – this requires enormous quantities of input, and as those quantities increase, quality tends to decrease.
Much of the most valuable training data is sensitive in nature (personally identifiable or proprietary information) and this poses major issues for both individuals wishing to protect their identity, and businesses who wish to control how their data is used. For AI operators, data quality is essential for inputs, therefore it is in their interest to collect as much valuable data as possible for their foundation model. As a result of this tension between the two, businesses must understand and define how they wish AI to interact with their publicly available data and how that will impact their operations in the short, medium and long term.
Data protection in an age of AI
In the short term, businesses must think now about how they wish their data to interact with AI scrapers and crawlers, which ingest large amounts of information to feed into large language models (LLM) to train and provide high-value outputs. If your business’ product is publicly available data, for example a news outlet, you must consider the implications of an AI ingesting your articles to train its model. For the past two years, this practice has been widespread.
Several legal challenges have been raised against the use of AI scrapers and crawlers without explicit permission. Mumsnet, the popular website forum aimed at parents, is taking legal action against OpenAI for ingesting user data via scrapers. Mumsnet is not as concerned with the fact that ingesting unverified data from users about pertinent health issues such as conception and pregnancy may mean AI outputs are based on false information. Instead, they’re more alarmed that ingesting this data would potentially lead to GenAI replacing Mumsnet entirely as a source of information on parenting in the longer term.
Many businesses may not be aware that AI tools are using their data to be trained on and provide outputs and instead lend ‘passive’ permission for their data to be scraped. The lack of explicit permissions given to AI tools makes protecting against these scrapers and crawlers even more difficult.
As a positive for AI operators, Google recently defeated a US federal class action lawsuit over the use of scrapers. The case was dismissed based on the fact the 143-page complaint was ‘meandering’ and too difficult to determine which causes of action were being alleged against Google. But these cases aren’t simple, and AI companies are likely to develop new scrapers and crawlers to bypass legal and technical roadblocks.
The legality of data scraping is just one element of the problem: storing it without permission is another. Clearview AI, a facial recognition firm, was fined $33 million and threatened with executive liability by the Dutch Data Protection Authority for building “an illegal database with billions of photos of faces” without appropriate consent. That said, the Australian Information Commissioner dropped legal action against Clearview AI for the same scraping and storing of billions of images. This contradictory regulatory approach between different jurisdictions highlights the challenges that businesses face when considering the deployment of GenAI for real-world use cases. the company in question has been lucky so far, but others may not be so; facing multiple, successful cases across jurisdictions that ultimately are unaffordable.
Data use or abuse will define the utility of AI
Businesses must consider how they wish to integrate AI into their business model over the medium or long term if the value of AI is increasingly undermined by either indiscriminate ingestion or poor-quality data. Simply put, a GenAI solution works on inputs and outputs. If the inputted data is overwhelmingly biased against women, the outputs could reflect this. This is because the ‘black box’ nature of GenAI means that predicting outcomes can be difficult, even for the AI engineers who built it. When AI ingests social media content, this is especially problematic given the sheer number of bots that amplify misinformation on platforms, which could lead the model to believe that there is a much broader consensus on a specific issue than there really is.
There’s also the potential for foundation model training and data ingestion to create a feedback loop which could undermine the deployment of GenAI entirely: as more online content is artificially generated and of questionable value, this will make up an increasing share of the ingested data of the next generation of foundation models – hindering their development at best, or significantly increasing instances of ‘hallucination’ at worst.
Many businesses that we regularly speak to, however, are already in the process of balancing the data protection risks of AI with the impetus to deploy it quickly and begin experimentation to align with business priorities – and they’re taking steps to try to achieve this safely. Controlling what types of prompts and language can be used can reduce the overall risk of using a foundation model. For instance, making sure a GenAI is not used for ‘fact checking’ or suggesting facts, can reduce the risk of hallucinations where a model ‘imagines’ something to be true when it isn’t.
Already, many businesses have implemented onshore (locally deployed) GenAI platforms that are secure, and the data is not ingested by the AI company to retrain its model. Meta’s Llama models are a good example of an open-source foundation AI which can be deployed locally and use Retrieval Augmented Generation techniques (RAG) on company data without there being a risk of further ingestion.
Options for businesses to protect themselves against intellectual property infringement by AI trainers are somewhat limited: adjusting privacy policies, applying blockers to prevent access to scrapers and crawlers, or using paywalls are not without their downsides. AI operators will continue to refine scraping technology, and paywalls often reduce the amount of traffic and exposure a company receives from its website. However, significant legal challenges may have a long-term impact on where and how AI operators can ingest data. It is down to lawmakers and interested parties to act and help shape the data protection regime around AI.
In the meantime, AI platforms will continue to scrape and ingest data from a huge breadth of sources that are unverifiable by users of the GenAI. Businesses will have to consider how they want AI to engage with the data they produce and how they manage the implementation of AI internally to ensure it provides as much value as possible. Putting up guardrails now, whilst AI is nascent, will set the tone for years to come.
This is an extract from 'The essentials of AI risk'. You can read the rest here.

Stay a step ahead in an increasingly complex and unpredictable world
Our consultants stay on top of the latest megatrends that influence how organisations are attacked, whether related to terrorism, criminality, war or cyber.
We document their analysis here. Be the first to see it.