
What's inside?
It’s clear that US technology companies are ‘betting the house’ on widespread and deep AI adoption, but assessing how long this might take is much more difficult.
Usually with new technologies analysts utilise a well-known framework for measuring how well and how widely that technology is being adopted – this is known as the ‘Hype Cycle’ and is released annually by the analytical and consulting firm Gartner. The Cycle evaluates whether a technology is being used effectively and if the hype outweighs effectiveness.
The Cycle typically indicates that for the first couple of years, a new technology is mostly hype. Everyone is talking about it, but few are actually operationalising it. Gartner currently places GenAI at the top of this curve. Over the next couple of years, what usually happens, according to previous iterations of the Cycle, is that a technology surpasses the peak in the hype cycle and starts to become useful. At this point, people may talk about it less as a different technology comes along, or the promised efficiencies from the new technology are never realised.
Consequently, it enters a period not necessarily of obscurity, but one where discussion of that technology is significantly reduced: this is known as the ‘Trough of Disillusionment’. The trough then leads to a period of stable adoption where people use the new technology to build new companies, functionality, capabilities, and value propositions. Applications of the new technology that are genuinely useful to people and generate value start to emerge. At this point, the technology becomes normalised and starts generating returns, reaching a balance between hype and effectiveness.
For GenAI, we are quite far from that stable part of the hype cycle. There are several reasons for this, which we’ll discuss later. Nevertheless, when we talk about the significance of these recent advances in transformer AI, GenAI, and other nascent forms of AI, it’s clear that we have unlocked a new type of technology likely to be as significant and impactful as the widespread adoption of the Internet in the 1980s and 1990s – but with extraordinary capabilities that can mimic or surpass the decision-making, communication styles, and even creative knowledge of humans. These extraordinary capabilities are likely to create extraordinary risks.
How AI works and the question of reliability
In the early stages of GenAI, the focus was not on neural networks but on probabilistic graphical models. These models learned transitions between states using graph-based representations instead of bio-inspired structures – the human brain. These statistical language models had already shown practical applications in the commercial sector during the 1990s.
The progress towards today’s GenAI has been a gradual and continuous advancement in the field of machine learning and neural networks. A neural network is effectively a combination of connected neurons that fire when exposed to chemicals in the brain, operating as ‘on and off’ switches. They are essentially binary. The neural networks that power GPT generative AI models are replications of that structure.
This is crucial for several reasons. Firstly, it represents a completely different approach to processing information compared to all the technology, computers, servers, and information systems that humans have created in the last 150 years. When you build a computer or an information system, you construct it according to certain rules established during the system’s creation. Engineers who built the system are fully aware of how it will react in any given situation because it follows specific rules written into the system. In effect, these types of systems are built by humans and will only ever operate in a way envisaged by the humans that built them.
The difference with GenAI and the use of deep learning and neural networks is that we don’t fully understand how they work. AI engineers describe AI foundation models as not ‘built’, but ‘grown’. They use this terminology because we don’t design the foundation model from bottom to top, understanding its inputs and outputs and designing algorithms to turn an input into an output.
Instead, we effectively set the conditions for a neural network to generate its own understanding of the world based on our desired extrinsic or intrinsic reward factors. It’s this new way of ‘growing’ information technologies instead of ‘building’ them which tends to create the most nervousness and uncertainty among engineers, corporations and governments.
AI explainability
For example, when we design a new generative AI model, we don’t fully understand how it can create Python code in response to a prompt – we just know that it does. We understand at a high level that it’s creating a kind of picture in a statistical way in a 3D space, enabling the GenAI to build sentences and represent the meaning of words and human intent when prompted. However, we don’t really understand how it does that or how it’s able to do it with such specificity – some of the AI’s capabilities, therefore, will not be fully explainable.
This leads us to the first major risk of AI: we cannot necessarily predict its output for any given input. Currently, we use the term “hallucinations” for this phenomenon. The idea that we can’t predict the outputs for any given input in GenAI creates a significant amount of risk for companies deploying it without appropriate guardrails to manage, review, and reduce this unpredictability. A significant area of research and investment currently is known as predictable or explainable AI. This phrase refers to an ecosystem of research focusing on deploying multiple systems, regulations, and various AI agents to create a foundation model that has the same power as current GenAI but can operate in a way that provides some level of predictability between input and output. We still have a long way to go before this area of research turns into an area of investment, which then becomes an area of product development available for corporations and organisations to use.
The reason why predictability and explainability are so important is that when using AI, there are usually very low levels of control over its output. The same argument can be made for human beings, but from a legal and regulatory perspective, we usually operate on the idea that humans are responsible for their actions, decisions, and judgments. Above those humans is a system run and managed by other humans who also need to take responsibility for the actions of individuals within that structure. This moral, social and legal structure is used to make people more predictable through responsibility and accountability.
For example, in health and safety law, if someone makes a mistake that causes a coworker to lose their life, that individual is responsible for their actions, errors, and omissions. The organisation they work for is also held responsible for not managing that individual correctly to ensure the right decisions are made in a predictable and explainable way. So, although humans are as unpredictable as modern GenAI foundation models, there is an established legal structure to hold humans accountable and create societal-level incentives that lead humans to operate within a range of outputs we deem acceptable based on a range of inputs. We do not currently have this capability with GenAI.
Another consideration is that if we are using GenAI to make decisions, we may be in a situation where, if challenged by a client, customer, supplier, or vendor about why a particular decision was made regarding their business or circumstances in relation to the organisation, there is no way for the organisation to fully explain why the AI made that decision. Without a better understanding and explainability of GenAI foundation models, this will continue to be a significant area of concern for most organisations.
Is the hype warranted?
Even though GenAI has been available for two years, it has not yet unlocked significant economic growth in most sectors of the economy. As The Economist has pointed out, as greater investment is pushed into GenAI-related companies and providers, the greater the amount of economic value that would need to be unlocked to meet these very high levels of investment, creating a value gap that may take many years for AI to fully close.
As we stated at the start, we believe we’re at the top of the Gartner Hype Cycle regarding GenAI. Over the long term, the impacts of AI on organisations are likely to be profound. From our perspective, when discussing AI with clients, the biggest risk is that organisations do not have a coherent, realistic, and persuasive AI strategy. The deployment of AI will create associated risks that need to be managed, treated, transferred, terminated, or tolerated, but the greatest risk remains in not deploying AI at all and not having a good understanding of how the organisation needs to be restructured based on the brand-new capabilities that GenAI presents.
In this discussion, we focus on GenAI, but clearly, there are many different types of AI. If we break down the terminology, AI is merely a system or infrastructure that can create human-like judgments and insights, which is not biological in nature. This includes GenAI, machine learning, and other types of reinforcement learning algorithms that in some way mimic the operation of the human brain. Over the years, it’s likely that we will see brand-new types of AI that we can’t even envisage currently.
The capabilities unlocked by AI are significant enough today to necessitate a complete re-evaluation of each organisation’s role in the economy and its relationship with clients and customers. Every organisation needs to assess the capabilities of AI, considering advancements likely to come into play over the next couple of years, to understand how it can be best deployed to unlock value through growth, increased revenue or cost reduction.
The risks of deploying and implementing forms of AI cannot override the impetus for organisations to avoid being left behind by the coming revolution that this type of technology will likely create.
How is AI going to change business in the long term?
Another consideration around the deployment of AI is a realistic understanding and evaluation of how well your senior leadership team understands technology and absorbs the likely coming changes to the economy. We tend to find when having discussions with clients that this is the first stumbling block to getting a coherent AI strategy off the ground.
When we look at the impacts that AI will have across the economy, it’s clear that it is not equal in all sectors or geographies. Current GenAI models are very good at summarising unstructured data and information but are unprepared to create information from nothing because they are prone to the hallucinations we have previously described. Businesses are understandably very reluctant to adopt GenAI in a widespread and blanket manner due to reliability issues.
Current AI foundation models also have questionable capability in engaging stakeholders outside the parameters of their modelling and have limited datasets. This is partially a safeguarding mechanism because of the uncertainty in how neural networks work. For instance, AI researchers found that when AI models ingested more open-source datasets the outputs became less ethical and more reflective of human bias.
Over time, as people become more comfortable with GenAI and the safeguards being put in place by foundation model developers like Google and Anthropic are gradually reduced, there is a danger that AI intelligence models will be provided direct access to the Internet, or at the very least, direct access to much larger data sources. This must be tightly controlled and legislated, as unintended consequences of black-box AI solutions will not only lead to liability questions, but also potentially create dangerous outcomes for humans.
Over the next two years, AI agents will become more common. An AI agent will enable companies to utilise GenAI to perform organic actions in their systems. For example, sending an email, requesting a document, or carrying out a review. These foundation model GenAIs will use specific function toolboxes to achieve tasks. It’s very likely that within five years, AI may be able to make and have calls with individuals to collect data and structure it in an established way.
This puts many jobs at risk both in back-office functions, but also the workload alleviation for higher skilled jobs may mean headcount also reduces across front-office roles. This massive overhaul of the workforce will require government intervention to both protect workers rights, but also ensure the transition is not uncontrolled. These regulations would fall into two areas; regulation of the foundation models themselves or the foundation model developers, and regulation in certain sectors where sector regulators, or even legislators at the national level, decide that certain jobs can only be performed by humans because of either their decision-making capacity or the criticality of those jobs and employment to the broader economy.
Focusing now on what society might look like because of the shift in the job market is critical to ensuring proportionate use of technology and will also help organisations develop and communicate a coherent strategy for how they will respond to an economy which may be transformed through the deployment of GenAI. Leadership teams need to bear in mind that strategic changes in their organisations may take years, while step-changes in the capabilities of AI can be released in months. Balancing short-term priorities with considering the long-term impacts of this kind of technology has always been difficult – but it’s likely to get a lot worse.
This is an extract from 'The essentials of AI risk'. You can read the rest here.
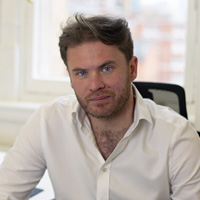

Stay a step ahead in an increasingly complex and unpredictable world
Our consultants stay on top of the latest megatrends that influence how organisations are attacked, whether related to terrorism, criminality, war or cyber.
We document their analysis here. Be the first to see it.